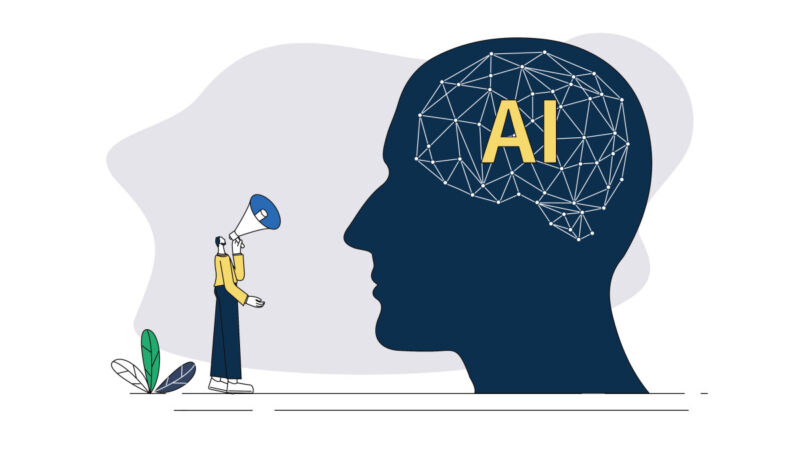
Getty Images
On Tuesday, Meta announced SeamlessM4T, a multimodal AI model for speech and text translations. As a neural network that can process both text and audio, it can perform text-to-speech, speech-to-text, speech-to-speech, and text-to-text translations for “up to 100 languages,” according to Meta. Its goal is to help people who speak different languages communicate with each other more effectively.
Continuing Meta’s relatively open approach to AI, Meta is releasing SeamlessM4T under a research license (CC BY-NC 4.0) that allows developers to build on the work. They’re also releasing SeamlessAlign, which Meta calls “the biggest open multimodal translation dataset to date, totaling 270,000 hours of mined speech and text alignments.” That will likely kick-start the training of future translation AI models from other researchers.
Among the features of SeamlessM4T touted on Meta’s promotional blog, the company says that the model can perform speech recognition (you give it audio of speech, and it converts it to text), speech-to-text translation (it translates spoken audio to a different language in text), speech-to-speech translation (you feed it speech audio, and it outputs translated speech audio), text-to-text translation (similar to how Google Translate functions), and text-to-speech translation (feed it text and it will translate and speak it out in another language). Each of the text translation functions supports nearly 100 languages, and the speech output functions support about 36 output languages.
In the SeamlessM4T announcement, Meta references the Babel Fish, a fictional fish from Douglas Adams’ classic sci-fi series that, when placed in one’s ear, can instantly translate any spoken language:
Building a universal language translator, like the fictional Babel Fish in The Hitchhiker’s Guide to the Galaxy, is challenging because existing speech-to-speech and speech-to-text systems only cover a small fraction of the world’s languages. But we believe the work we’re announcing today is a significant step forward in this journey.
How did they train it? According to the Seamless4MT research paper, Meta’s researchers “created a multimodal corpus of automatically aligned speech translations of more than 470,000 hours, dubbed SeamlessAlign” (previously mentioned above). They then “filtered a subset of this corpus with human-labeled and pseudo-labeled data, totaling 406,000 hours.”
As usual, Meta is being a little vague about where it got its training data. The text data came from “the same dataset deployed in NLLB,” (sets of sentences pulled from Wikipedia, news sources, scripted speeches, and other sources and translated by professional human translators). And SeamlessM4T’s speech data came from “4 million hours of raw audio originating from a publicly available repository of crawled web data,” of which 1 million hours were in English, according to the research paper. Meta did not specify which repository or the provenance of the audio clips used.
Meta is far from the first AI company to offer machine-learning translation tools. Google Translate has used machine-learning techniques since 2006, and large language models (such as GPT-4) are well known for their ability to translate between languages. But more recently, the tech has heated up on the audio processing front. In September, OpenAI released its own open source speech-to-text translation model, called Whisper, that can recognize speech in audio and translate it to text with a high level of accuracy.
SeamlessM4T builds from that trend by expanding multimodal translation to many more languages. In addition, Meta says that SeamlessM4T’s “single system approach”—a monolithic AI model instead of multiple models combined in a chain (like some of Meta’s previous audio-processing techniques)—reduces errors and increases the efficiency of the translation process.
More technical details on how SeamlessM4T works are available on Meta’s website, and its code and weights (the actual trained neural network files) can be found on Hugging Face.