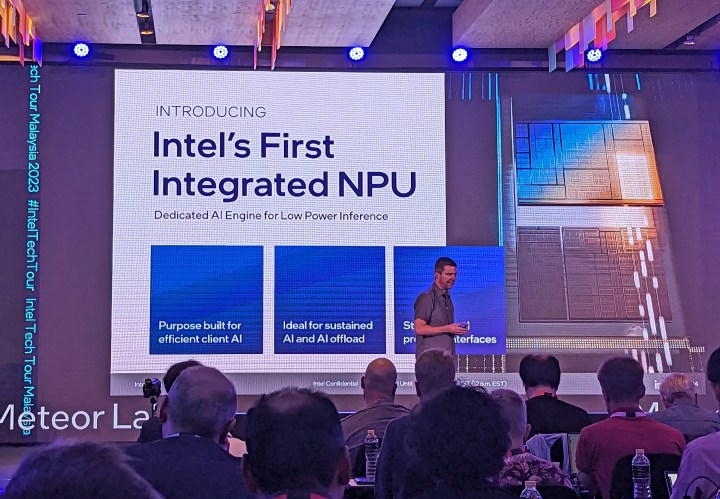
The AI era is upon us. With generative AI continuing to push forward, companies like Intel, AMD, and Qualcomm are also talking about the hardware side of the equation. With the introduction of the NPU, which stands for neural processing unit, processes that use AI will be sped up — at least, in theory.
Apple has been using an NPU in its chips for years now, so they aren’t exactly brand new. And yet, being heralded as the “next big thing” across various industries, they’re more important than ever.
What is an NPU?
At its core, an NPU is a specialized processor explicitly designed for executing machine learning algorithms. Unlike traditional CPUs and GPUs, NPUs are optimized for handling complex mathematical computations integral to artificial neural networks.
They excel in processing vast amounts of data in parallel, making them ideal for tasks like image recognition, natural language processing, and other AI-related functions. For example, if you were to have an NPU within a GPU, the NPU may be responsible for a specific task like object detection or image acceleration.
NPU vs. GPU vs. CPU: Understanding the differences
While GPUs (graphics processing units) are proficient at parallel processing and are often used in machine learning, NPUs take specialization a step further. GPUs are versatile and excel in handling graphics rendering and parallel tasks, while CPUs (Central Processing Units) are the general-purpose brains of a computer, handling a wide range of tasks.
NPUs, however, are purpose-built for accelerating deep learning algorithms. They are tailored to execute specific operations required for neural networks. This degree of specialization allows NPUs to deliver significantly higher performance for AI workloads compared to CPUs and even GPUs in certain scenarios.
GPNPU: The fusion of GPU and NPU
The concept of GPNPU (GPU-NPU hybrid) has emerged, aiming to combine the strengths of GPUs and NPUs. GPNPUs leverage the parallel processing capabilities of GPUs while integrating NPU architecture for accelerating AI-centric tasks. This combination aims to strike a balance between versatility and specialized AI processing, catering to diverse computing needs within a single chip.
Machine learning algorithms and NPUs
Machine learning algorithms form the backbone of AI applications. While often mistaken for AI, machine learning can be seen as a type of AI. These algorithms learn from data patterns, making predictions and decisions without explicit programming. There are four types of machine learning algorithms: supervised, semi-supervised, unsupervised, and reinforcement.
NPUs play a pivotal role in executing these algorithms efficiently, performing tasks like training and inference, where vast datasets are processed to refine models and make real-time predictions.
The future of NPUs
We’re seeing NPUs pop up all over the place in 2024, with Intel’s Meteor Lake chips being the most prominent. As to how big of a deal they will be in the future, that’s yet to be seen. In theory, enhanced AI capabilities will lead to more sophisticated applications and improved automation, making it more accessible across various domains.
From there, the demand for AI-driven applications should continue to surge, with NPUs standing at the forefront. Their specialized architecture, optimized for machine learning tasks, allows NPUs to forge forward in the world of computing. The fusion of GPNPUs and the advancements in machine learning algorithms will undoubtedly drive advancements we’ve not seen before, fueling the progression of technology and reshaping our digital landscape.
Right now, NPUs might not seem like a huge deal for most people, only speeding up things you can already do on your PC, like blurring the background in a Zoom call or performing AI image generation locally on your machine. In the future, though, as AI features come to more and more applications, they may be destined to become an essential part of your PC.
Editors’ Recommendations